特邀报告
简介:
会议邀请了香港理工大学章伟雄教授、卫宁健康首席科学家/人工智能 实验室主任陈旭教授级高工、浙江大学医学院附属邵逸夫医院林辉主任、中国科 学技术大学周少华教授等四位著名专家分别做题为 “Network-based genome-wide association studies of complex traits and diseases”、“医 疗领域中的大语言模型—研发到落地的实践探索”、“数字化助推医疗新生态” 和“生成式人工智能在医学影像的探索”的特邀报告。
特邀报告1
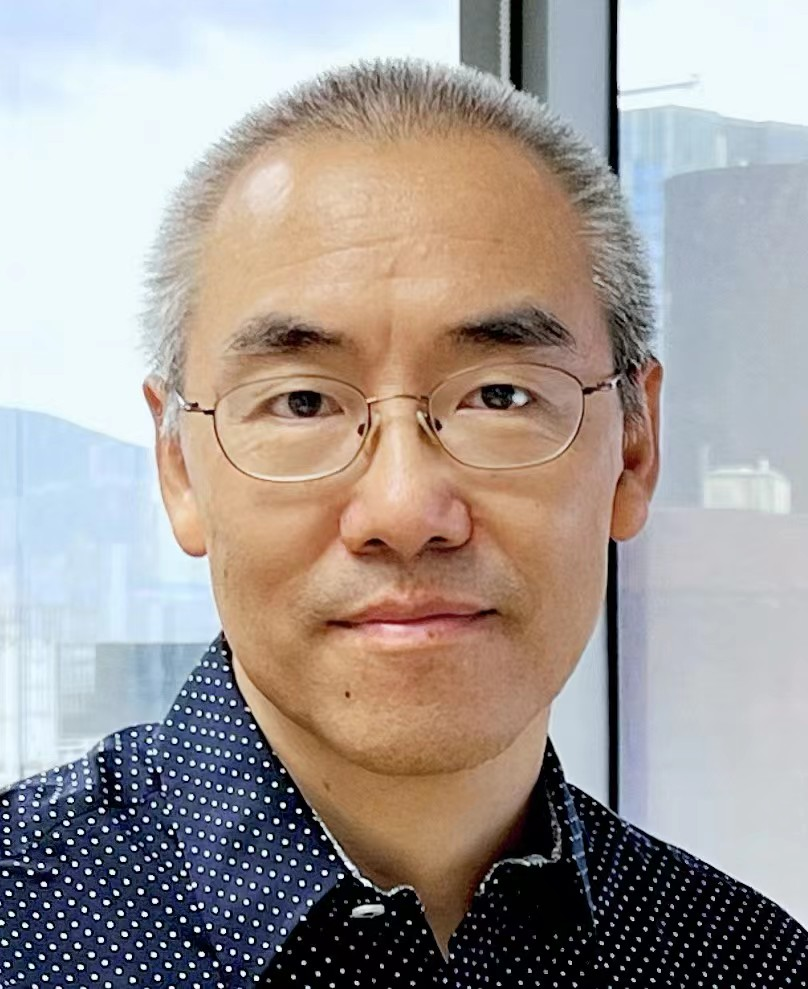
章伟雄教授 香港理工大学
Network-based genome-wide association studies of complex traits and diseases
时间:10月28日(周六),09:00-10:00
章伟雄教授: 拥有计算机学士(清华大学)和博士(美国加州大学洛杉矶分校 UCLA)学位,曾任美国圣路易斯华盛顿大学计算机和遗传学教授二十余年。 目前担任香港理工大学生物信息学与整合基因组学讲座教授和香港全球杰出创 科学人教授 (Hong Kong Global STEM Professor) 。
报告摘要: Hundreds of genetic biomarkers have been identified in complex disease studies, yet the bulk of “missing heritability” remains alarmingly elusive, which hinders the reliable diagnosis and effective treatment of complex diseases such as cancers and brain disorders. Combinatorial interactions ong multiple genomic loci and genetic heterogeneity in the human populations constitute candidate sources of the “missing heritability”. In this talk, I will first discuss the biological and computational challenges to the discovery of polygenic (combinatorial) biomarkers. I will then present a novel approach to network-based, allele-specific genome-wide association studies (GWAS) for systematic identification and analysis of polygenic biomarkers of complex traits and diseases. I will also present some of the results of the new method on psoriasis (an autoimmune skin disease) and the genomic structures of world populations of different ethnic backgrounds. I will discuss some ongoing research and new directions for future develoent for the identification of polygenic biomarkers of complex diseases, particularly along the line of deep-learning and graph techniques for large network analysis.
特邀报告2
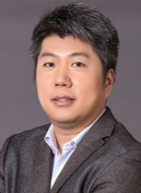
陈旭教授级高工 卫宁健康
报告题目:医疗领域中的大语言模型—研发到落地的实践探索
时间:10月28日(周六),10:15-11:15
陈旭教授级高工: 都柏林大学生物医学工程与信息学博士,卫宁健康首席科学家, 人工智能实验室主任,中国医学装备协会人工智能学组专委,中国医学影像 AI 产学研联盟理事。旅居欧洲十几年,长期从事欧洲医疗信息化标准,医疗信息建 模,医疗大数据挖掘和基于人工智能的医疗系统等研究工作。先后带领卫宁健康 人工智能团队获得多项国内外的奖项,2023 年带领团队研发了卫宁健康医疗领 域大模型 WiNGPT,基于 WiNGPT 的医护智能助手 WiNEX Copilot,以及 WiNEX 产品与解决方案,并于 10 月的卫宁健康 Winning World 2023 大会上 发布。卫宁健康 2022 年参加多项 CHIP 算法比赛并获的 2 个冠军 1 个季军。
报告摘要: 随着大型语言模型在医疗领域的不断涌现,其在医疗多方面展现出巨 大应用潜力。本报告将深入探讨医疗大模型的研发历程和实际应用的实践经验, 主要包括目标设定、核心功能、模型训练、数据资源、评估方法及应用能力。特 别地,我将集中讨论我们研发的第二代医疗大语言模型 WiNGPT 与分享产品落 地过程中的经验与教训。WiNGPT 旨在融汇医学知识、信息和数据,为医疗界提 供问答、诊断支持及知识传播服务。该模型整合了医学问答、自然语言理解和多 轮对话功能,覆盖八大医疗场景的 18 个子场景,满足 32 类医疗任务需求。最 后,我将从医疗信息科技从业者的角度分享对未来医疗技术发展的思考和展望。
特邀报告3
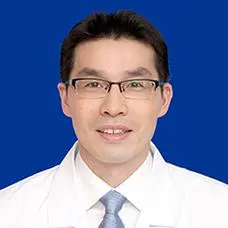
林辉教授 浙江大学医学院
报告题目:数字化助推医疗新生态
时间:10月29日(周日),08:30-09:30
林辉教授: 医学博士,浙江大学医学院附属邵逸夫医院,普外科,主任医师,互联 网与人工智能办公室主任。2002 年 7 月毕业于浙江大学医学院临床医学系,同年 攻读浙江大学外科学硕士,2005 年提前攻读博士,师从我国著名微创外科专家蔡秀 军教授,2007 年 7 月获外科学博士学位,同年进入邵逸夫医院从事普外科临床、科 研及教学工作。作为技术骨干参与支架法无缝合空腔脏器吻合技术的设计和相关实 验研究,为实现无缝合空腔脏器吻合新技术及其吻合支架的临床应用提供可靠的研 究数据和实践依据。作为技术骨干参与设计开展乳腺癌术后腹腔镜带蒂网膜瓣乳房 重建术,并建立了一套相对完整的技术体系,填补了国内技术空白。擅长肝胆、胃 肠及腹外疝等疾病的诊治,熟练掌握腹腔镜为主的微创外科技术,对肝脏肿瘤及微 创保胆技术有深入研究。
报告摘要: 数字化开启文明新纪元。数字技术已成这个时代最重要的变革力量。 数字技术如何改变医疗健康?围绕这个问题,报告者立足医疗场景分享自身在互 联网医疗、医疗人工智能和数字疗法领域的思考和初步探索,从组织、供给和服 务形式三个维度呈现数字化视角下医疗健康的未来。
特邀报告4
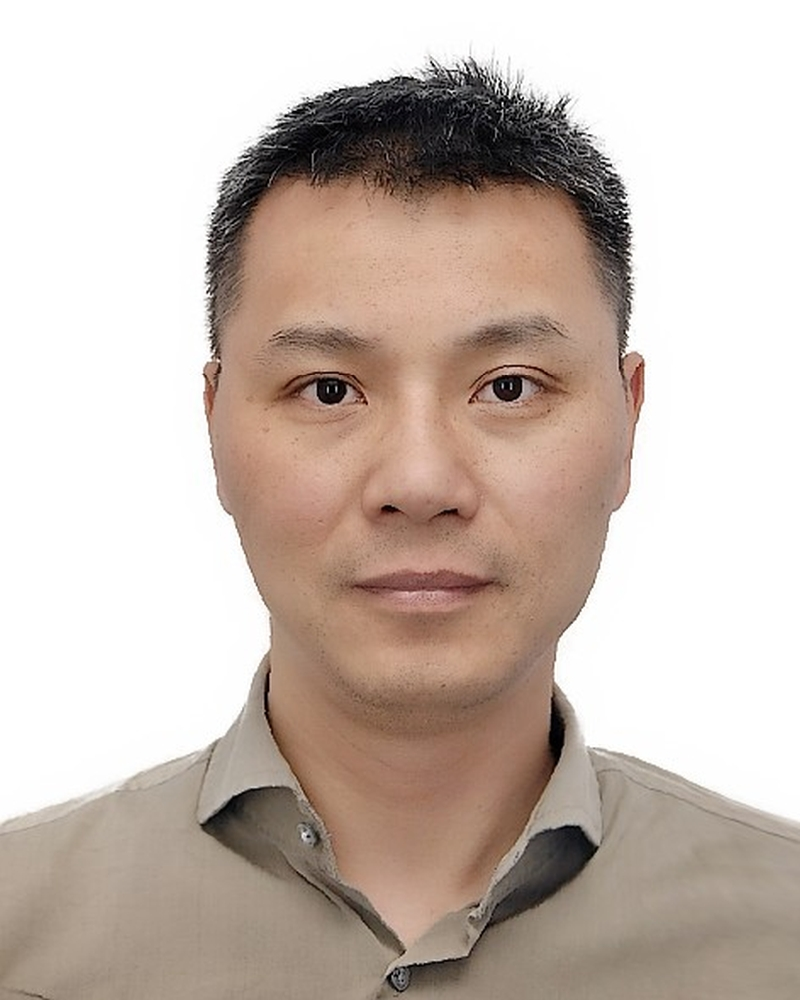
周少华教授 中国科技大学
报告题目:生成式人工智能在医学影像的探索
时间:10月29日(周日),14:00-15:00
周少华教授: 周少华教授是中国科学技术大学讲席教授,博导,生物医学工程学院 执行院长,医学影像智能与机器人研究中心主任。长期致力于医学影像的研究创新、 应用落地及学术服务。在医学影像领域,率先开展了“机器学习+知识模型”的系统 性研究,最近明确了“大任务、小数据”的研究范式和挑战,探出了标注高效、通 用模型、知识融合的三大解决途径。发表 270 余篇学术论文和章节,谷歌学术总引 用超 14000 次,H 因子为 64;撰编学术专著 8 本。在工业界有长达 14 年的经历, 曾任西门子高级研发总监及首席 AI 专家。获授权专利逾 140 项,算法成功转入 10 多项 FDA 批准的产品。产品部署在全球几千家医院,用于逾 7 百万病人的临床治疗 诊断。担任行业顶级协会 MICCAI 司库兼理事、Medical Open Network for AI ( MONAI )顾问、顶级期刊 Medical Image Analysis 、 IEEE Trans. Pattern Analysis and Machine Intelligence ( TPI ) 、 IEEE Trans. Medical Imaging(TMI)等编委、顶级会议 AAAI、CVPR、ICCV、 MICCAI 和 NeurIPS 等领域主席、MICCAI2020 的程序联席主席、《视觉求索》公 众号联席主编。他多次因算法、论文、专利、服务等多次获得认可和奖励,包括 MICCAI 年轻科学家奖提名文章、RD100 科技奥斯卡奖、西门子年度发明家、马里 兰大学 EE 杰出校友奖、BMEF 年度编辑、Fellow of IEEE、AIMBE (美国医学与生 物工程院)、NAI(美国国家学术发明院)等。
报告摘要: Medical images are widely used in clinical decision making and artificial intelligence (AI) technologies are commonly utilized in medical imaging and image analysis. In this talk, we address the aspect of medical imaging and present an overview of how AI facilitates the generation of medical image through recovery and synthesis. Medical image recovery attempts to recover the original image under adverse imaging conditions, such as metal artifacts, slow acquisition time, etc. Medical image synthesis attempts to synthesize, from an acquired image under current conditions, a novel image under different conditions. We will cover three neural approaches: (i) Dual domain network (DuDoNet) for metal artifact reduction in CT via joint learning in both sinogr and image domains and MR image reconstruction from underspled k-space data via joint and recurrent learning in both frequency and image domains; (ii) Causal image synthesis (CIS) for counterfactually synthesizing MR brain images in 3D via the nontrivial leverage of a causal graph and 3D StyleGAN; and (iii) Unified multimodal image synthesis (UMIS) for imputing missing MR images of multiple modalities from any combination of available ones with a single unified model. Our recovery and synthesis approaches leverage deep neural networks as cores, integrate specific domain knowledge, and achieve high quality images.